In the digital age, cybersecurity remains a paramount concern for organizations worldwide. As cyber threats evolve in complexity and stealth, traditional security measures struggle to keep pace. Enter Machine Learning (ML), a subset of Artificial Intelligence (AI) that is transforming the cybersecurity landscape. This blog delves into the cutting-edge machine learning techniques revolutionizing threat detection, offering a beacon of hope in the escalating cyber warfare. We will also explore the intriguing applications of ML in AI chatbot development services, generative AI in healthcare, and personalized learning, demonstrating the multifaceted value of machine learning in enhancing cybersecurity postures.
The Evolution of Cyber Threats and ML’s Response
Cyber threats have undergone a significant evolution, becoming more sophisticated and harder to detect. Traditional security systems, reliant on predefined rules and signatures, falter against novel and intricate attacks. Machine learning, with its ability to learn from data, identify patterns, and make decisions with minimal human intervention, offers a dynamic solution. ML algorithms, through continuous learning, adapt to the ever-changing threat landscape, providing a proactive and predictive approach to cybersecurity.
Anomaly Detection: The Watchdog of Cybersecurity
One of the most potent ML techniques in threat detection is anomaly detection. This approach involves training ML models on normal network behavior and then monitoring for deviations that could indicate a security breach. The strength of anomaly detection lies in its ability to flag unusual activities, from subtle changes in network traffic to irregular user behaviors, which could often go unnoticed by traditional security systems. By identifying these anomalies in real-time, organizations can swiftly respond to potential threats, mitigating risks and preventing damage.
Predictive Analytics: The Clairvoyant Defender
Predictive analytics is another frontier where ML is making significant strides in cybersecurity. By analyzing historical data and identifying correlations and patterns, ML models can forecast potential security incidents before they occur. This preemptive insight allows organizations to bolster their defenses in anticipation of attacks, staying one step ahead of cybercriminals. Predictive analytics exemplifies the shift from reactive to proactive cybersecurity strategies, emphasizing prevention rather than cure.
AI Chatbot Development Services: The Unsung Heroes of Cybersecurity
While not immediately associated with cybersecurity, AI chatbot development services are emerging as valuable allies in the fight against cyber threats. These advanced chatbots can serve as the first line of defense, engaging with users to detect phishing attempts, disseminate security alerts, and guide individuals through secure online practices. By integrating ML into chatbot frameworks, these virtual assistants can learn from interactions, continuously enhancing their ability to identify and respond to potential security risks.
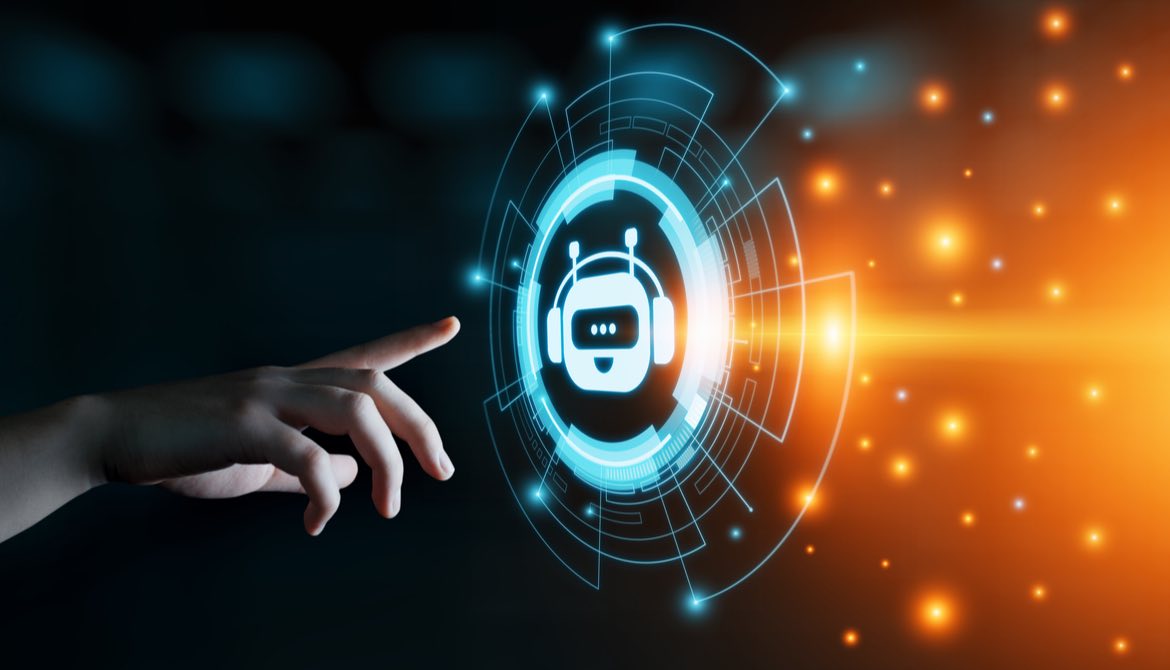
Generative AI in Healthcare: A Double-Edged Sword
Generative AI, known for its ability to create new data and simulations, finds a unique application in healthcare cybersecurity. By generating realistic cyber-attack simulations, healthcare organizations can test and improve their security systems, preparing them for actual threats. However, the power of generative AI also poses a risk if leveraged by cyber attackers to create sophisticated phishing campaigns or malware. Balancing the innovative use of generative AI with robust security measures is crucial in safeguarding sensitive healthcare data.
Personalized Learning: Empowering the Human Firewall
The human element often remains the weakest link in cybersecurity. Here, personalized learning platforms, powered by ML, come into play. These platforms can tailor cybersecurity training to individual users’ learning patterns and knowledge levels, enhancing their awareness and ability to recognize threats. By fostering a well-informed workforce, organizations can strengthen their human firewall, making it a formidable barrier against cyber attacks.
Navigating the Challenges: Ethical Use and Data Privacy
The integration of ML in cybersecurity, while promising, is not without challenges. Concerns around the ethical use of AI, data privacy, and the potential for AI-driven security tools to be exploited by malicious actors necessitate a cautious approach. Ensuring transparency, securing ML algorithms against tampering, and adhering to data protection regulations are essential steps in leveraging ML for cybersecurity without compromising ethical standards or privacy.
The Future Landscape: ML-Driven Cybersecurity
The future of cybersecurity is inextricably linked to the advancements in machine learning. As ML techniques become more sophisticated, their potential to detect and thwart advanced cyber threats will continue to grow. Organizations that embrace these ML-driven security solutions will not only enhance their defense mechanisms but also foster an innovative and adaptive security culture capable of withstanding the cyber challenges of tomorrow.
Enhancing Incident Response with Machine Learning
The rapid identification of cyber threats is only one piece of the puzzle; an effective incident response is equally crucial. Machine learning is revolutionizing this aspect by automating the analysis of incidents, prioritizing threats based on their severity, and suggesting the most effective remediation strategies. This not only speeds up the response times but also ensures that cybersecurity teams can focus their efforts on the most critical issues. Incorporating machine learning into incident response protocols enhances an organization’s resilience against attacks, turning reactive measures into a strategic, proactive defense.
The Synergy of Machine Learning and Blockchain for Secure Transactions
In an era where digital transactions are omnipresent, ensuring their security is paramount. The convergence of machine learning with blockchain technology presents a formidable solution. Machine learning algorithms can monitor blockchain transactions for unusual patterns that might indicate fraudulent activity, while the inherent security features of blockchain provide an immutable record, enhancing trust and transparency. This synergy not only bolsters the security of digital transactions but also opens new avenues for secure, AI-enhanced blockchain applications across various sectors.

Fostering a Culture of Continuous Learning and Adaptation
The dynamic nature of cyber threats demands a continuous learning approach, not just for AI systems but also for the human elements in cybersecurity. Personalized learning platforms equipped with machine learning can provide cybersecurity professionals with up-to-date training on the latest threats and defense strategies, fostering a culture of continuous learning and adaptation. This ensures that both AI systems and their human counterparts are always at the forefront of cybersecurity innovation, ready to tackle emerging threats with the latest knowledge and techniques.
Conclusion
Machine learning is revolutionizing the field of cybersecurity, offering advanced techniques for detecting and preventing cyber threats. From anomaly detection to predictive analytics, ML empowers organizations to adopt a proactive stance in safeguarding their digital assets. The integration of ML in areas such as AI chatbot development services and personalized learning further underscores its value in enhancing cybersecurity measures. As we navigate this digital era, the fusion of machine learning and cybersecurity emerges as a cornerstone of digital resilience, heralding a new age of fortified defenses against the cyber adversities of the future.